The Ultimate Guide to Data Labelling Tools: Enhance Your AI Projects
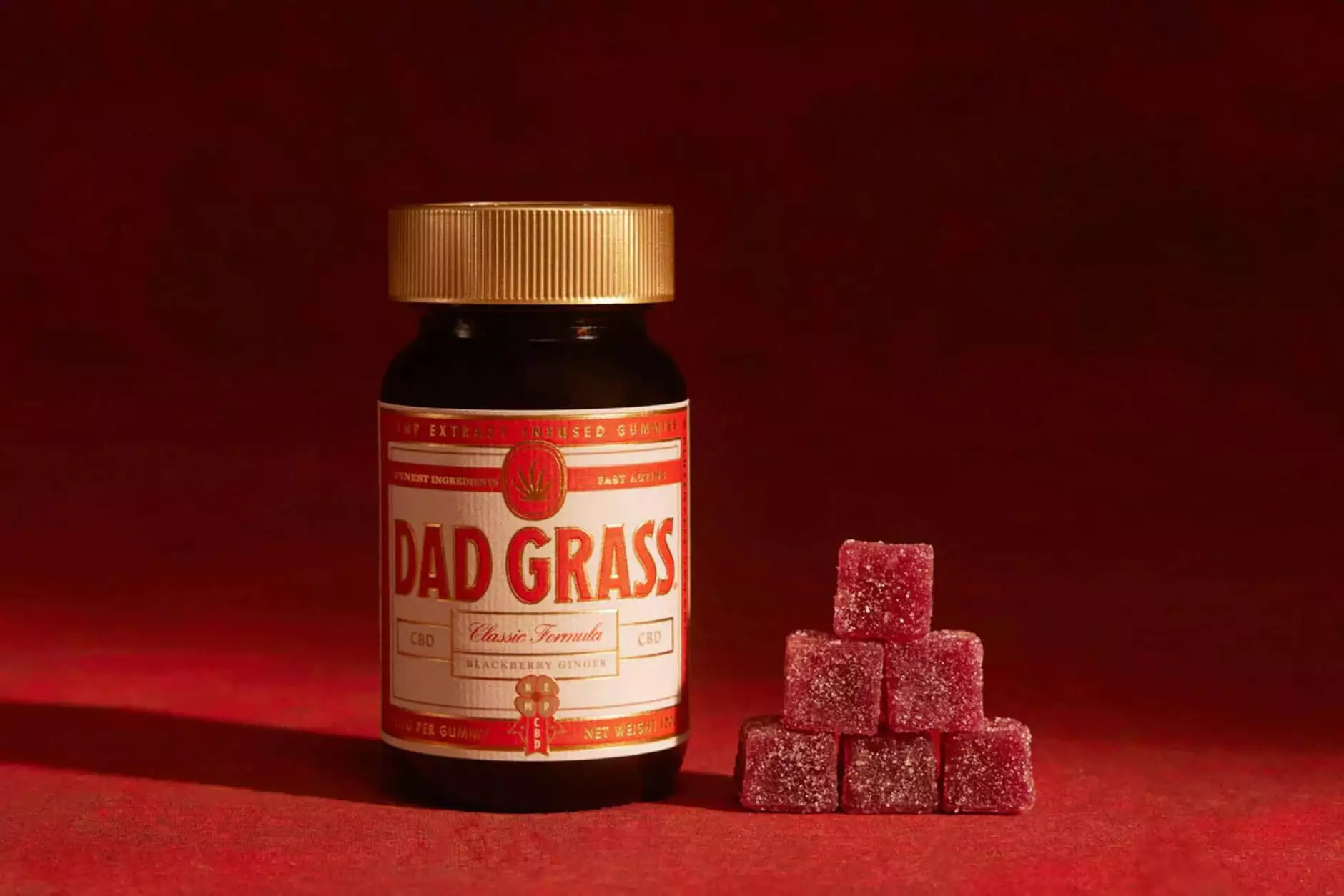
In the ever-evolving landscape of artificial intelligence, the significance of data cannot be overstated. Data labelling tools play a crucial role in the success of AI models by ensuring that datasets are accurately annotated, enabling machines to understand and learn from them effectively. In this comprehensive guide, we will delve deep into what data labelling tools are, their importance, and how businesses can leverage them for better outcomes, especially focusing on the offerings from keylabs.ai.
Understanding Data Labelling Tools
Data labelling, also referred to as data annotation, is the process of categorizing and tagging data for use in machine learning and artificial intelligence. Data labelling tools provide a platform where this process can be conducted efficiently and accurately.
- Types of Data Labelling Tools: Data labelling tools can be categorized based on the type of data they handle, including images, text, audio, and videos.
- User Interface: Some tools offer highly intuitive interfaces that make the labelling process easier, while others may require advanced technical skills.
- Automation Features: The best data labelling tools include automated features that can accelerate the labelling process.
Why Data Labelling is Critical for AI/ML Success
For machine learning algorithms to function correctly, they require meticulously labelled data. Here’s why using data labelling tools is vital:
1. Improved Model Accuracy
Accurate data labelling directly impacts the performance of machine learning models. Quality labelled data leads to better interpretation and understanding, which ultimately results in more precise predictions.
2. Time Efficiency
Manual data labelling can be a time-consuming process. However, using advanced data labelling tools streamlines this process, allowing businesses to save time and allocate resources effectively.
3. Scalability
As projects grow, the amount of data that needs labelling can increase exponentially. Data labelling tools enable businesses to scale their labelling efforts to keep up with project demands.
4. Quality Control
Data labelling tools often include review mechanisms that ensure high-quality labels. This is vital for maintaining the integrity of data and the overall success of AI projects.
Key Features of a Good Data Labelling Tool
When choosing a data labelling tool, it is essential to look for features that can meet your specific needs. Here are some must-have attributes:
- User-Friendly Interface: The best tools prioritize user experience, offering simple navigation and clear instructions.
- Robust Editing Features: Tools should provide users with the ability to easily edit and refine labels.
- Customizable Workflows: Different projects may require different processes; customizable workflows allow flexibility in handling varied labelling tasks.
- Integration Capabilities: A good data labelling tool can seamlessly integrate with other AI development tools and platforms, simplifying your workflow.
- Collaboration Options: The tool should enable teams to work together effectively, sharing insights and feedback during the labelling process.
Exploring keylabs.ai: A Leading Data Annotation Platform
Keylabs.ai stands out in the crowded market of data annotation platforms. By focusing on efficiency, usability, and flexibility, keylabs.ai offers an exceptional suite of features designed to enhance the data labelling process. Here’s a comprehensive look at what makes keylabs.ai a go-to solution for businesses.
1. Wide Range of Data Support
Keylabs.ai supports various data types including images, text, audio, and video. This versatility makes it ideal for businesses that work with different kinds of data in their machine learning projects.
2. Advanced Machine Learning Integration
The platform’s capability to integrate with leading machine learning frameworks enables users to transition seamlessly from data labelling to model training. This ensures a cohesive workflow that can markedly enhance productivity.
3. High-Quality Annotation
Keylabs.ai employs a combination of automated and manual labelling methods. This hybrid approach not only speeds up the labelling process but also guarantees high-quality annotations through rigorous quality assurance procedures.
4. Collaboration and Project Management Tools
The platform includes robust project management tools that facilitate collaboration among multiple stakeholders. Team members can easily track progress, share feedback, and ensure that everyone is aligned towards common goals.
5. Scalability and Flexibility
Keylabs.ai is built to accommodate projects of varying sizes, making it a scalable solution for businesses aiming for growth. Whether you have small datasets or massive volumes of data, the platform can handle your needs efficiently.
Choosing the Right Data Labelling Tool for Your Business
When selecting a data labelling tool, consider these critical steps:
1. Assess Your Business Needs
Determine the specific requirements based on the type of data you work with and the scale of your projects. If your projects involve numerous data types, look for a versatile tool like keylabs.ai.
2. Evaluate Tool Features
Look for the essential features discussed earlier. Conduct trials or demos where possible to see the tool’s interface and functionalities in action.
3. Analyze Budget Constraints
While it’s vital to choose a tool that meets your needs, cost-effectiveness should also be a consideration. Compare various options and choose one that offers good value for the features provided.
4. Read Reviews and Testimonials
Seek feedback from existing users of data labelling tools. Online reviews and case studies can provide insight into the effectiveness of a tool in real-world applications.
Best Practices for Effective Data Annotation
Even with excellent data labelling tools, following best practices is essential for successful data annotation:
- Start with Clear Guidelines: Develop precise annotation guidelines to ensure consistency among all labellers.
- Use Qualified Labelers: Ensure that the individuals or teams performing the labelling have a clear understanding of the data and the task requirements.
- Regular Quality Checks: Implement a system for continuous quality assurance to identify and correct errors promptly.
- Iterate and Improve: Use feedback from AI model performance assessments to refine the labelling process continuously.
Conclusion: Unlocking AI Potential with Data Labelling Tools
Investing in high-quality data labelling tools is a vital step for businesses looking to harness the power of artificial intelligence. Platforms like keylabs.ai provide robust solutions that streamline the annotation process and enhance data quality. By following best practices and leveraging advanced features, organizations can not only improve their AI outcomes but also maintain a competitive edge in their respective industries. The future of AI is data-driven, and with the right tools, businesses can unlock its full potential.