Harnessing Machine Learning for Enhanced Business Analytics
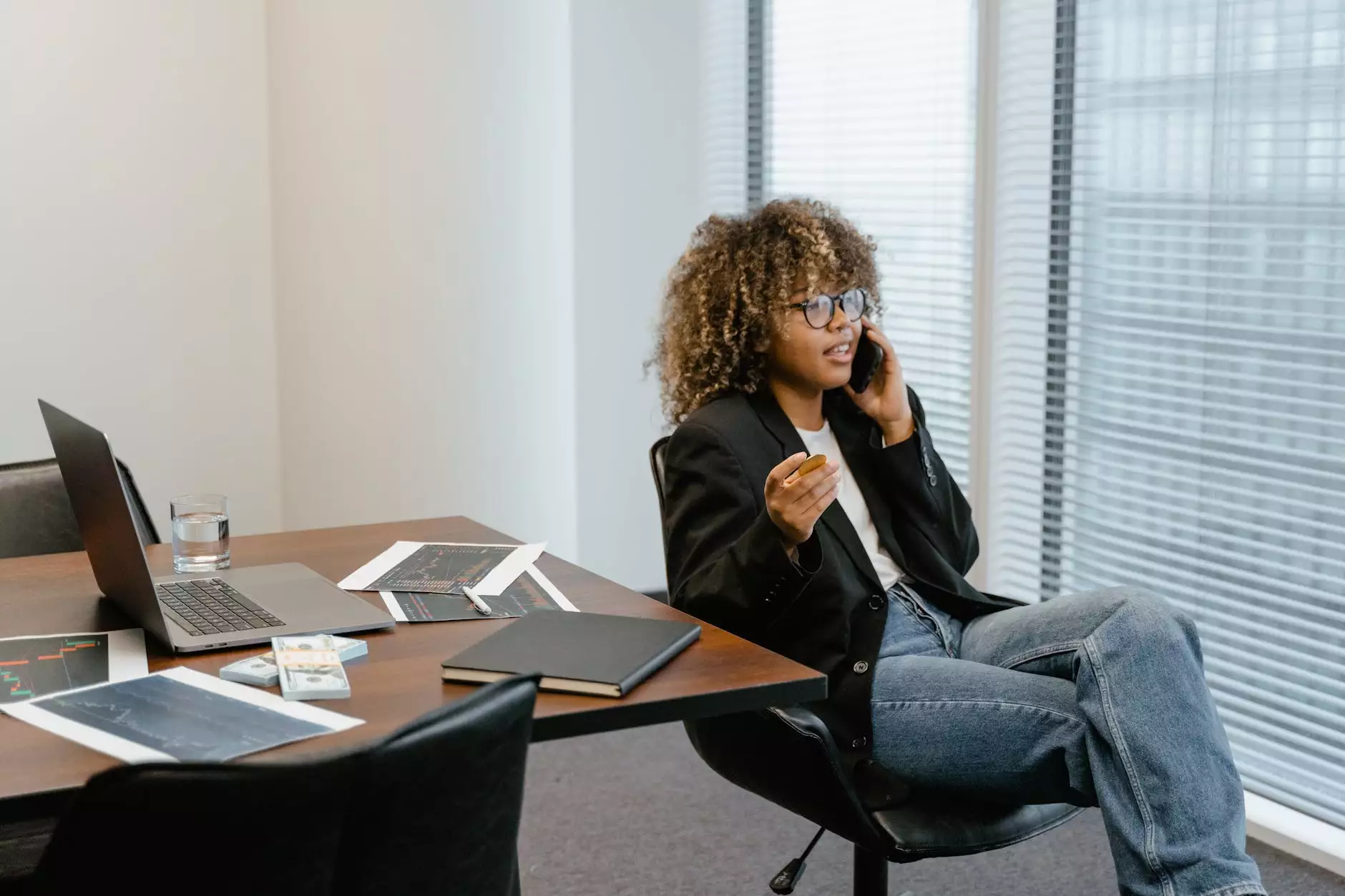
In the rapidly changing landscape of modern business, organizations are continually seeking ways to enhance their operational efficiency and improve decision-making. One of the most impactful technological advancements in this area is machine learning for analytics. This innovative approach leverages algorithms and statistical models to enable systems to learn and make data-driven predictions, ultimately leading to informed decision-making.
What is Machine Learning for Analytics?
Machine learning (ML) refers to a subset of artificial intelligence that focuses on the development of algorithms that allow computers to learn from and make predictions based on data. In the context of analytics, machine learning utilizes data inputs to generate insights that can significantly influence business strategy and operations.
These insights can range from identifying customer trends to predicting sales patterns. By integrating machine learning into analytics, businesses can process vast amounts of data efficiently, uncover hidden patterns, and gain predictive insights that are critical for success.
The Role of Machine Learning in Business Analytics
Organizations today are inundated with data from various sources. To remain competitive, they must harness this data effectively. Machine learning plays a pivotal role in this process by transforming raw data into actionable intelligence. Here’s how:
1. Enhancing Data Processing Capabilities
Machine learning algorithms can process large datasets far more quickly than traditional methods. This capability allows businesses to:
- Analyze real-time data: Businesses can gain insights and make decisions based on the most current data available.
- Improve data accuracy: Machine learning can enhance the integrity of predictions by identifying and correcting errors in datasets.
- Automate routine analyses: Data scientists can focus on more strategic tasks while ML algorithms handle data processing.
2. Driving Predictive Analytics
One of the most significant advantages of machine learning for analytics is its ability to provide predictive insights. By analyzing historical data, machine learning models can identify trends and patterns, enabling businesses to forecast future outcomes. This ability is invaluable in various domains, such as:
- Sales Forecasting: Predictive analytics can help businesses forecast sales by analyzing historical sales data alongside market trends.
- Customer Behavior Prediction: Understanding consumer behavior allows companies to tailor their marketing efforts.
- Risk Management: Financial organizations can anticipate potential risks and take proactive measures to mitigate them.
3. Improving Decision-Making Processes
The insights provided by machine learning can greatly enhance decision-making. By using data-driven insights, businesses can make informed choices that lead to better outcomes. Key benefits include:
- Reduced Uncertainty: Data-driven insights allow decision-makers to base their strategies on concrete evidence rather than intuition).
- Increased Efficiency: Streamlined processes can significantly improve productivity.
- Strategic Insights: Companies can focus on long-term strategies informed by predictive analytics.
Applications of Machine Learning for Business Analytics
Machine learning can be applied across various business segments to enhance operations. Here are some prominent applications:
1. Customer Insights
Understanding consumer needs is crucial for any business. Machine learning algorithms can analyze customer data to deliver personalized experiences, segment customers, and identify trends, leading to targeted marketing strategies and improved customer satisfaction.
2. Supply Chain Optimization
Machine learning can enhance supply chain efficiency through better demand forecasting, inventory management, and route optimization. By predicting customer demand accurately, businesses can reduce overstock and stockouts, saving costs and improving service levels.
3. Fraud Detection
Fraud detection has become increasingly important. Utilizing machine learning algorithms, businesses can analyze transactional data to detect anomalies and fraudulent activities. This proactive approach helps reduce financial losses and enhance trust with customers.
Machine Learning in Content Management Services
In the realm of content management services, machine learning can significantly improve how businesses handle their content repositories. By automating categorization and enhancing search functionalities, organizations can streamline content retrieval.
1. Automating Content Tagging
Machine learning algorithms can analyze content to automatically generate relevant tags, enhancing the organization of digital assets and improving content discoverability.
2. Personalized Content Delivery
By analyzing user behavior and preferences, machine learning can facilitate personalized content delivery, ensuring that users receive the most relevant information.
Business Process Automation Services Enhanced by Machine Learning
Combining machine learning with business process automation services can lead to significant productivity improvements. Automated processes can learn from previous data inputs to refine their operations continuously.
1. Workflow Optimization
Machine learning can analyze workflow processes to identify inefficiencies, enabling businesses to streamline their operations and reduce costs.
2. Intelligent Process Automation
By integrating machine learning with traditional automation, businesses can build intelligent systems capable of adapting to changing conditions without human intervention.
The Importance of Data Governance in Machine Learning
As organizations adopt machine learning for analytics, effective data governance becomes essential. Businesses must ensure that the data they are using is accurate, secure, and compliant with regulations.
1. Ensuring Data Quality
High-quality data is critical for the success of machine learning models. Implementing robust data governance practices ensures that data is clean and reliable.
2. Regulatory Compliance
With increasing regulations around data privacy, such as the GDPR, businesses must ensure their data practices comply with legal standards. This aspect of data governance is non-negotiable in using machine learning for analytics.
Future Trends in Machine Learning for Business Analytics
The future of machine learning is bright, with ongoing advancements promising even greater capabilities. Key trends include:
1. Enhanced Real-Time Analytics
As technology evolves, the ability to perform real-time analytics will dramatically improve, allowing businesses to act on insights instantaneously.
2. Increased Collaboration Between Human Intelligence and Machine Learning
The future will likely see a blended workforce where humans and machines collaborate to achieve superior outcomes.
3. Wider Adoption of Automated Machine Learning (AutoML)
AutoML platforms are emerging, enabling non-experts to create machine learning models, thus democratizing access to powerful analytics capabilities.
Conclusion
In conclusion, the integration of machine learning for analytics is not just a trend but a necessity for businesses looking to thrive in today's competitive landscape. From enhancing decision-making processes to driving operational efficiency, the benefits are vast. As organizations like Intalio embrace these technologies, they position themselves for long-term success in an increasingly data-driven world.
Investing in machine learning capabilities, alongside robust data governance practices, will empower businesses to harness the full potential of their data, ensuring they remain agile and competitive in the future.